Stratification Can Reduce Statistical Power
To ensure all arms in a randomized clinical trial have roughly equal percentages of participants from key subgroups, statisticians often stratify patient assignment to each arm and account for these stratification factors in the final analysis. A computational study led by researchers with the SWOG Cancer Research Network has found that for modestly sized phase 2 trials, stratified analysis with more than one or two stratification factors can significantly reduce the power of a trial to detect a positive result.
The work will be presented at the American Society for Hematology (ASH) 2022 Annual Meeting and Exposition, on December 12 in New Orleans (abstract #4027).
To test the effect of stratification on statistical power, the researchers simulated trial data using design assumptions from the protocol of a randomized phase 2 acute myeloid leukemia trial that was being finalized. They ran multiple simulations based on the design, using increasing numbers of stratification factors in randomization and in analysis. They then estimated the statistical power for each simulation while keeping the allowable type-1 error roughly constant. Also known as a false positive, a type-1 error concludes an effect when no effect actually exists.
The researchers found that for the small phase 2 trial design they worked from, which had a total sample size of 84 participants (42 per arm), a stratified analysis that included one or two stratification factors did not significantly decrease the statistical power from that of an unstratified design. When four or six stratification factors were used, however, statistical power decreased from around 88 percent in the unstratified setting to 75 percent or 55 percent, respectively. Generally, clinical trial designs aim to have at least 80 percent power to detect a difference between treatment arms.
The analysis was led by Anna Moseley, a SWOG biostatistician based at the Fred Hutchinson Cancer Center. Moseley noted there is currently a lack of concrete data in the literature on the impact of additional stratification factors on statistical power.
“The impetus for the experiment was that there weren’t any data to look at when trying to advise study teams on how many factors to use in stratification, at least not in the setting of a phase 2 trial – so with a small sample size – with potentially many stratification factors.”
The new findings, she said, “can help statisticians give more informed advice when designing trials, and a justification for limiting our number of stratification factors to those that are going to really make a difference in how likely each patient is to meet the primary endpoint.”
“There might be a tendency to assume that we want the arms to be as equal as possible,” she added, “which makes sense, because you want all of these important factors to be distributed equally across the arms to make the trial most likely to be unbiased, but there is a drawback to that: it will hit your power to show a positive result.”
The SWOG Cancer Research Network is a cancer clinical trials group funded by the National Cancer Institute (NCI), part of the National Institutes of Health (NIH). The analysis was supported by the NIH/NCI through grants CA180888 and CA180819.
The author team also included Boris Freidlin, of the NCI Division of Cancer Treatment & Diagnosis, Biometric Research Program; Rory Shallis, and Amer Zeidan, both of Yale University School of Medicine; David Sallman, of Moffitt Cancer Center; Rich Little, of the NCI, Cancer Therapy and Evaluation Program (CTEP); Harry Erba, of Duke University School of Medicine, Duke Cancer Institute; and Michael LeBlanc and Megan Othus, both of the SWOG Statistics and Data Management Center and the Fred Hutchinson Cancer Center.
Other Recent Stories
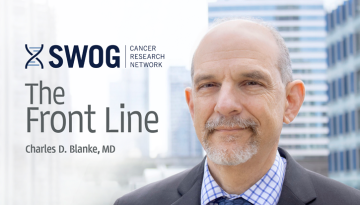
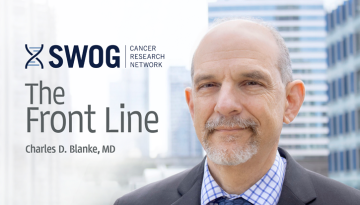
